The Semantic Systems Biology Approach
What is Systems Biology?
There are many definitions of systems biology, ranging from: \”a research approach that seeks to describe the overall behavior of a biological system through detailed, quantitative experimentation combined with conceptual or computational modeling of the systems components and their interactions\” (c.f. SysBioSIG), to the very concise: \”the study of biological function that derives from interactions\”.
What is Semantic Systems Biology?
Semantic technologies are playing an increasingly important role in capturing and modeling biological knowledge. Semantic systems biology can complement the bottom-up approach with data-driven generation of hypotheses. Therefore, Semantic Systems Biology (SSB) is a systems biology approach that uses semantic description of knowledge about biological systems to facilitate integrated data analysis.
What are the features of a Semantic Systems Biology approach?
As is depicted in the figure to the right, biological knowledge is gathered and integrated into a knowledge base; clockwise: (02:00) Data are checked for consistency, and querying and automated reasoning leads to (05:00) hypotheses which in turn help to design experiments. (07:00) The experimentation generates new data and might also verify hypotheses. (10:00) The data generated are afterwards integrated into the knowledge base, establishing a cyclical process. The workflow of Semantic Systems Biology approach is similar to Kitano’s original depiction of Systems Biology [H. Kitano (2002), Systems biology: a brief overview, Science 295: 1662-4]: two main phases exist in an iterative process, corresponding to a computational, data processing phase (top half of the cycle) and an experimental, wet-lab phase (bottom half of the cycle). The hypothesis formulation in this new paradigm is enabled by the technologies behind knowledge representation, Semantic Web, automated reasoning and querying described above. Kitano’s Systems Biology paradigm hinges on model-based hypothesis generation and validation in new experiments, iteratively leading to model refinement. In Semantic Systems Biology, data and new knowledge are (automatically) checked for consistency with existing knowledge, and queries and automated reasoning on a genome wide knowledge resource are exploited to infer new hypotheses on model architecture.
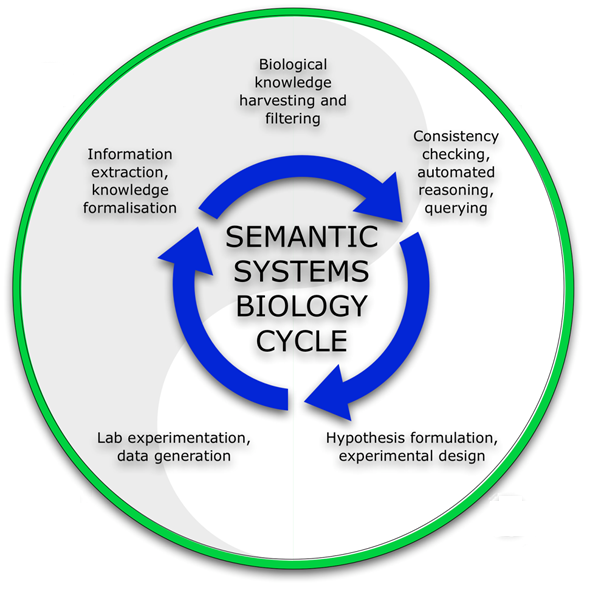
There are some key elements in this new paradigm:
- Knowledge representation
- Data integration
- Reasoning → Hypothesis
- Querying → Hypothesis
Team Members
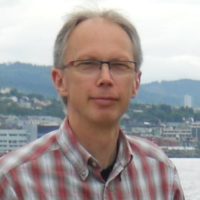
Martin Kuiper
Head of the group www.ntnu.edu/employees/martin.kuiper druglogics.eu/profile/martin-kuiper
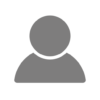
Vladimir Mironov
Systems engineer for the BioGateway resources.
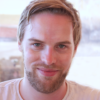
Stian Holmås
Responsible for developing the Cytoscape App and web resources.
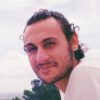
Rafael Riudavets
Responsible for use case studies and quality assurance.
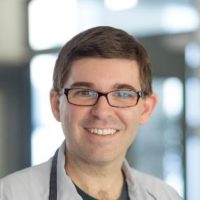
Marcio Luis Acencio
Department of Clinical and Molecular Medicine (IKOM), Norwegian University of Science and Technology (NTNU)
druglogics.eu/profile/marcio-luis-acencio/
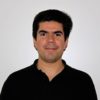
Erick Antezana
Affiliated researcher, main initiator of BioGateway and the semantic systems biology work.
Currently working at Bayer Cropscience, Ghent, Belgium
Alumni
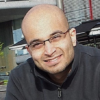
Aravind Venkatesan
Literature services group, European Bioinformatics Institute (EMBL-EBI)
www.ebi.ac.uk/about/people/aravind-venkatesan
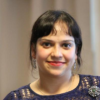
Konika Chawla
Bioinformatics core facility (BioCore), NTNU
Ph.D. Systems Biology, NTNU www.ntnu.no/ansatte/konika.chawla
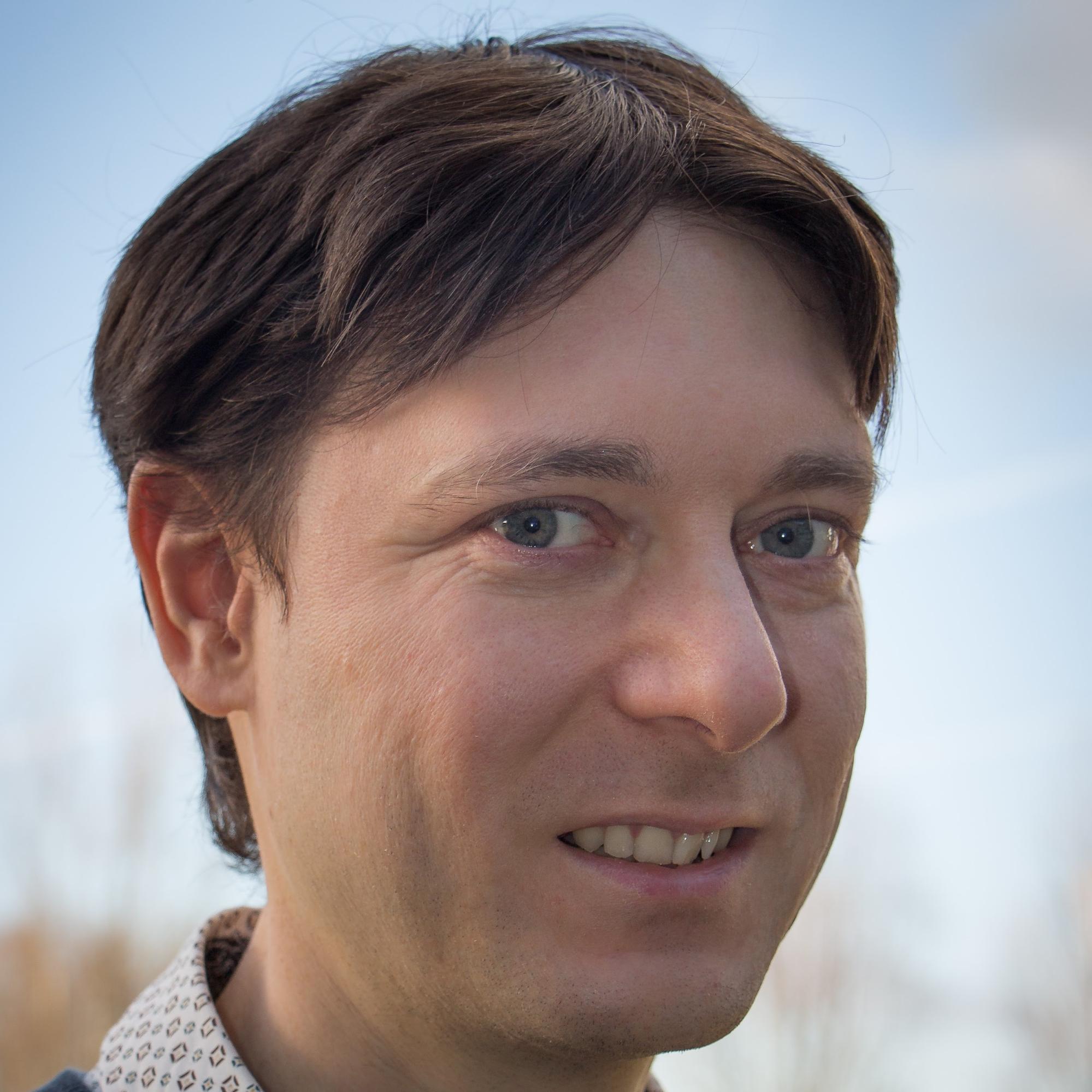
Ward Blondé
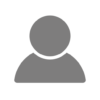
Jens Hollunder
Responsibilities and work areas
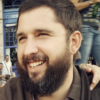
Mikel Egaña Aranguren
Torres Quevedo Fellow at Eurohelp Consulting, Spain
mikel-egana-aranguren.github.io/
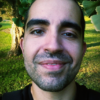
Alejandro Sanz de Galdeano Varela
www.linkedin.com/in/asdgvarela/
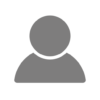
Mario Prieto
http://www.cbgp.upm.es/index.php/es/informacion-cientifica/csbgp/bioinformatica
Collaborations
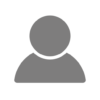
Jin-Dong Kim
Responsibilities and work areas